The dramatic performance advantage of Graphcore IPU systems is on display once again with the latest round of MLPerf comparative benchmarks.
Graphcore’s Bow Pod16 turned in a 31% faster time-to-train than NVIDIA’s more expensive flagship DGX-A100 640GB on ResNet-50.
On BERT, a 37% improvement over the previous MLPerf round ensures that Graphcore systems remain a leader on price/performance for the popular language model also.
In the first ever third-party submission on a Graphcore system, Baidu achieved the same performance for BERT training using its PaddlePaddle software framework; demonstrating the ease of adoption that is driving rapid growth in the IPU ecosystem.
When Graphcore entered the MLPerf process in 2021, we were responding to calls to demonstrate the IPU’s capabilities on (relatively) long-established measures of AI compute performance.
Our customers and industry commentators, reasonably, wanted to see that the IPU’s dramatically differentiated architecture could deliver alongside the vector processors that ResNet and BERT were, literally, built on. We’ve done that... and then some.
Beyond these useful numbers, we are focusing on the successors to ResNet and BERT that Graphcore customers are now deploying, bringing greater levels of accuracy and efficiency.
Emerging model classes such as Graph Neural Networks (GNNs) take full advantage of the IPU’s MIMD architecture, its ability to support fine-grained parallelism, and other AI-centric characteristics. The performance differential for these types of workload is – in some cases – an order of magnitude or greater, compared to the vector processor architectures that make up most of today’s AI compute.
ResNet-50
A combination of our new Bow IPU and software refinements has reduced time-to-train on ResNet-50 by up to 31%, compared to the previous round of MLPerf (v1.1).
While performance jumps up across the board, the price of Bow Pod systems remains the same as their predecessors, resulting in a huge perf/$ advantage.
Customers typically cite Graphcore’s ability to deliver much more AI computing power for their money as one of their motivations for choosing IPU systems.
The performance advantage is now even more pronounced against significantly higher priced systems, such as the DGX-A100 640GB.
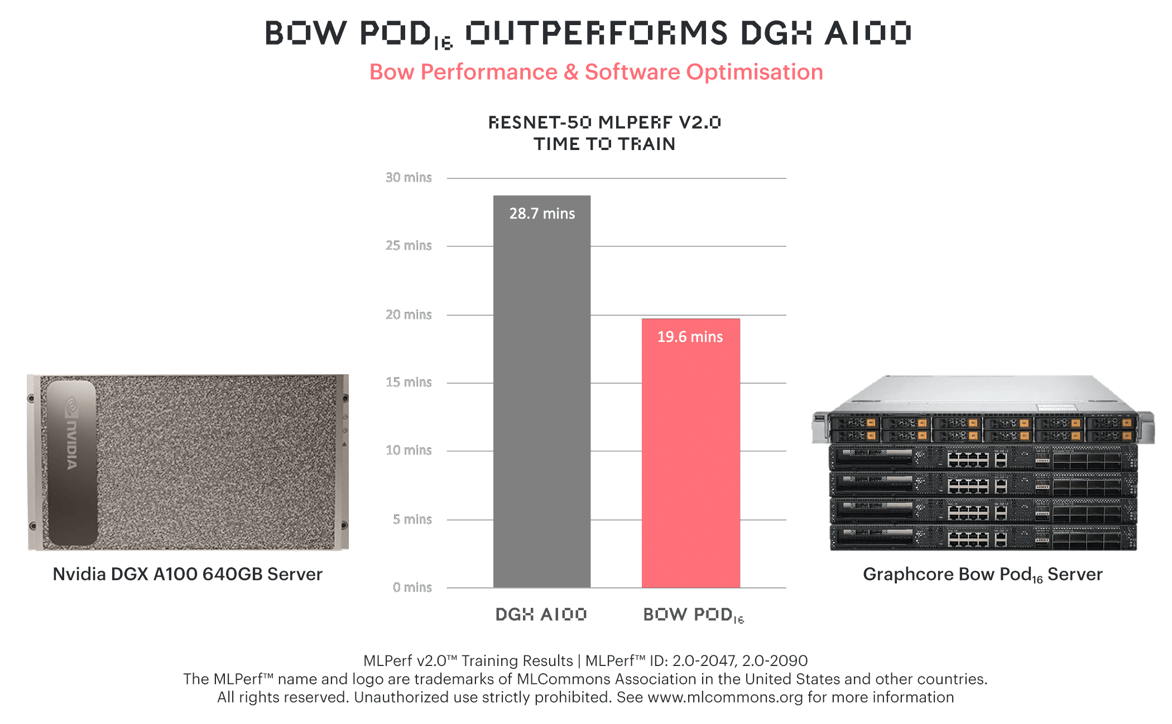
BERT and Baidu
Time-to-train improvements of up to 37% on BERT translate to a significant price/performance advantage for Graphcore.
Baidu’s PaddlePaddle submissions using Bow Pod16 and Bow Pod64 to train BERT show results on par with Graphcore’s own submission using PopART.
Not only is this a powerful third-party verification of Bow Pod performance, it demonstrates the flexibility of Graphcore systems that is driving rapid growth in the IPU ecosystem. Baidu was able to integrate Poplar with its widely used PaddlePaddle software framework and deliver stellar results.
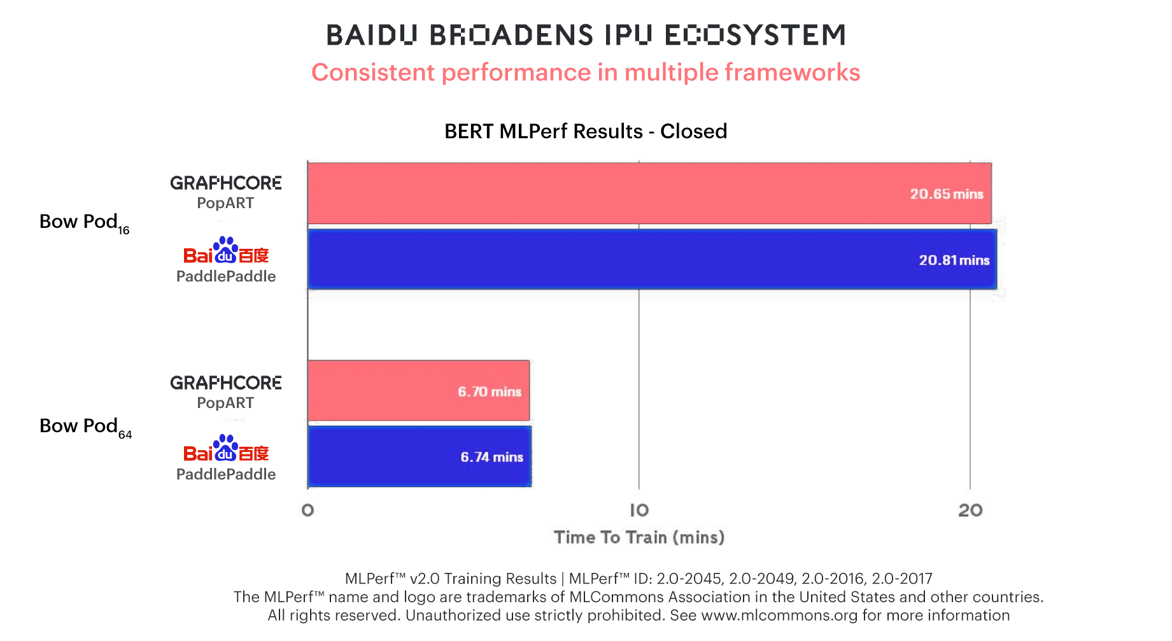
RNN-T
Graphcore also submitted results for RNN-T in the open category.
Recurrent Neural Network Transducers are a sophisticated way of performing highly accurate speech recognition. They are widely used on mobile devices because of the ability to deploy the trained model locally on handsets for minimum latency.
In this case, Graphcore focused on RNN-T training, in partnership with our customer, Gridspace, which provides voice solutions spanning customer service, compliance and process automation.
The RNN-T model was trained on 700GB or 10,000 hours of speech and scaled to run on a Bow Pod64, reducing training time from weeks to days.
Our submission on an RNN-T to MLPerf 2.0 reflects Graphcore’s customer-centric approach to implementing and optimizing models for the IPU.
Beyond MLPerf
Beyond ResNet, BERT and RNN-T, the vast majority of IPU-ready models available in Graphcore’s Model Garden have been driven by customer demand.
We are supporting increasingly sophisticated models that take advantage of the IPU’s made-for-AI MIMD architecture, including its ability to run thousands of truly independent program threads
One such example was outlined recently by Twitter’s Head of Graph ML Research, Michael Bronstein, who showed IPUs delivering up to 10x performance gains for Temporal Graph Networks.
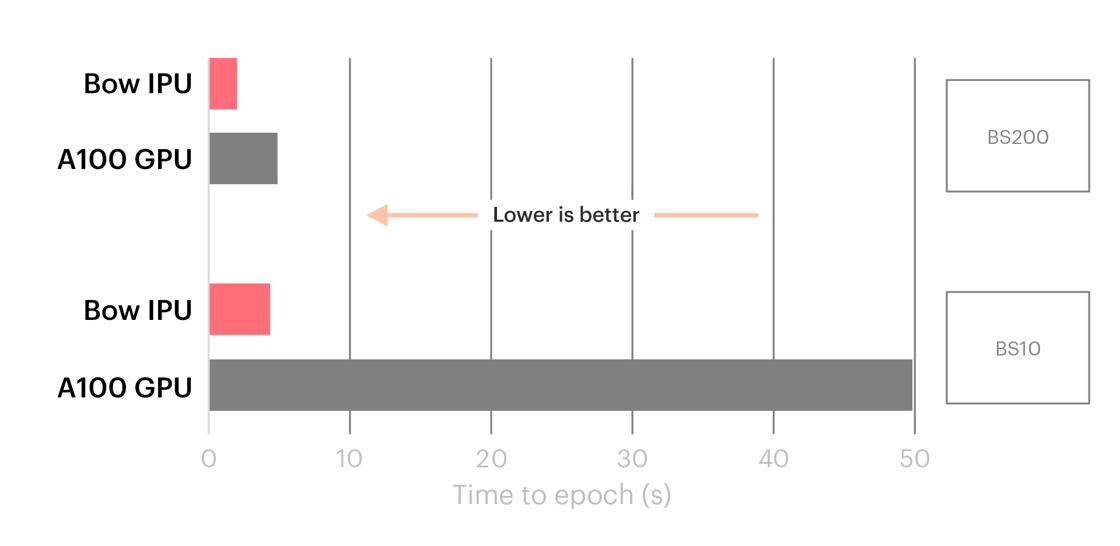
IPUs outperformed GPUs for TGNs, with the difference especially pronounced for common, smaller batch sizes.
Likewise, the US Department of Energy’s PNNL lab reported 36x faster time-to-result on SchNet GNN using an IPU Classic system, compared to a V100 GPU setup.
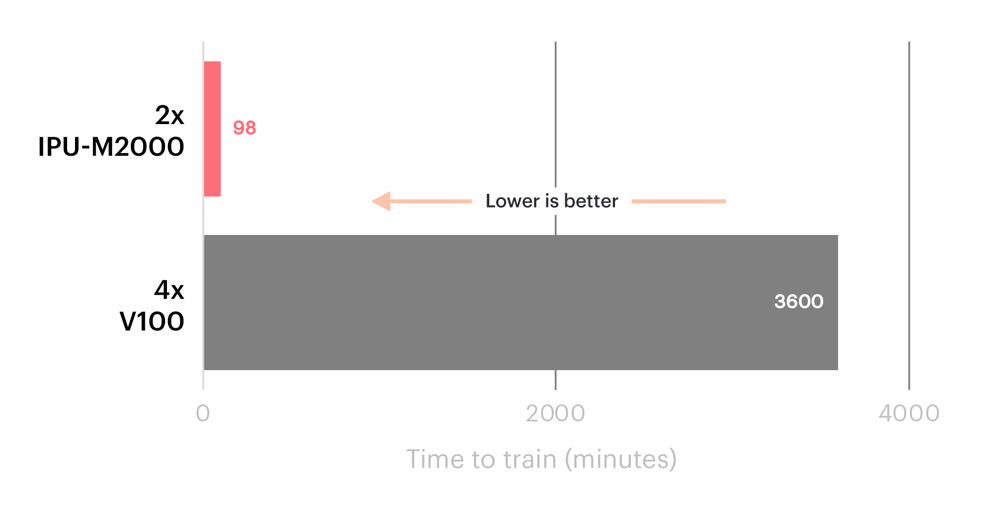
SchNet GNN performance on IPU Classic vs V100 GPUs.
In addition to the significant advantage seen for GNN use cases, IPU-friendly techniques such as conditional sparsity and mixture-of-experts, are increasingly making their way out of the research lab and into the wider world.
AI Computing, Evolved
As well as bringing new capabilities and greater levels of accuracy, next generation models need to operate more efficiently if AI is to continue progressing at its current rate. That, in turn, demands the sort of compute platform offered by Graphcore.
Model size and complexity has grown, in a few short years, from hundreds of millions of parameters to billions and now trillions.
That has given us unprecedented functionality – including multi-modal models able to handle text, speech, vision and more.
However, the rate of growth is vastly outpacing the capacity of any silicon to keep pace. Instead, the industry has, for several years, been simply throwing more compute at the problem – an unsustainable solution.
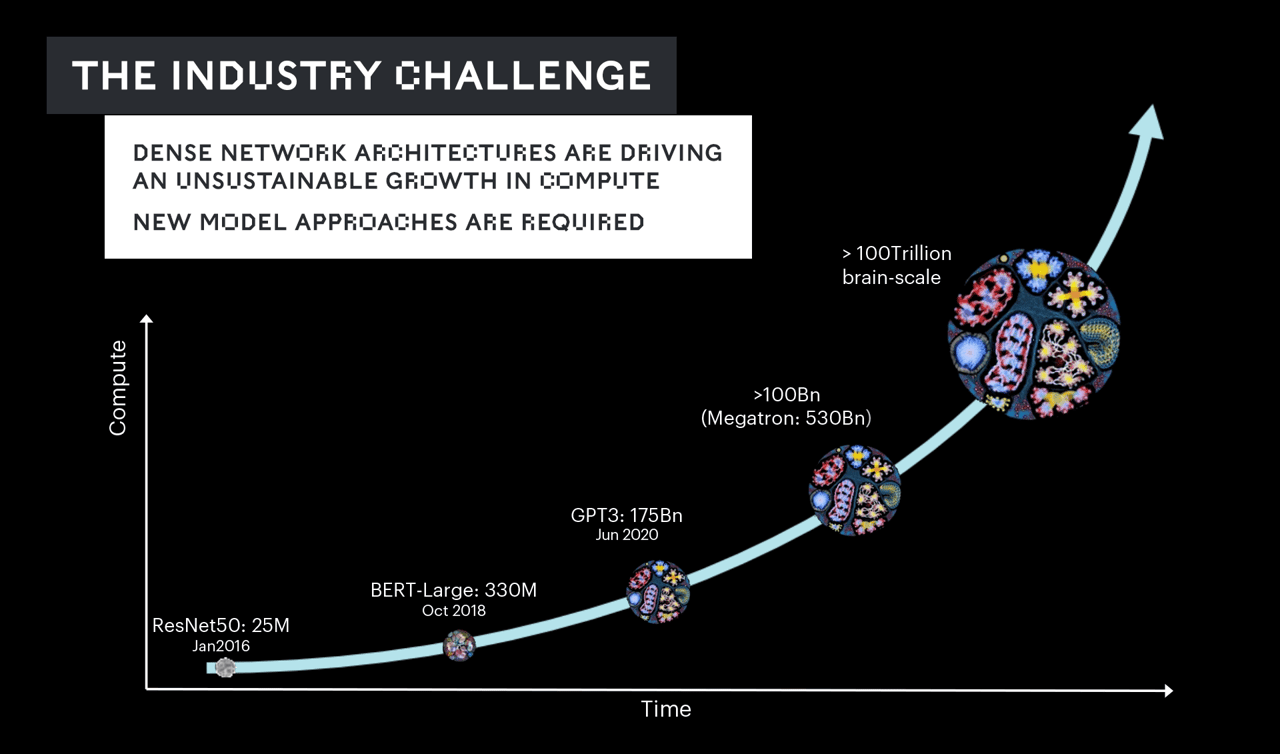
We face the very real prospect of a world where training the largest models could require millions of processors working for months on end, costing billions of dollars or more.
Avoiding this “compute crunch” and ensuring the continued advancement of AI will require advanced models to be developed on, and optimized for, systems like the IPU.
This is what Graphcore is engaged in and where, with our customers and partners, we are already seeing results.
We recently announced plans to work with Aleph Alpha on its large multi-modal models, using next-generation techniques enabled by the IPU, to deliver greater computational efficiency.
The need for large AI compute systems won’t go away, but with the IPU’s highly differentiated approach it will be possible to reach multi-trillion parameter scale with much more affordable systems.
To this end, we are developing The Good Computer, which will take advantage of next generation IPU processors, as well as new approaches to system memory and mass storage.
The Good Computer is more than the next step in Graphcore’s product roadmap; it represents a sustainable way forward for AI compute and the many beneficial applications that will bring.
WATCH: Computers for superhuman cognition – Simon Knowles at AICAS 2022.