Today, we are excited to announce a partnership between the U.S. Department of Energy’s Pacific Northwest National Laboratory (PNNL) and Graphcore that ushers in a new era in advancing the use of AI for scientific research in several disciplines.
PNNL is a leading center for scientific discovery in chemistry, data analytics, Earth and life sciences, and for technological innovation in sustainable energy and national security. This collaboration has already started to break new ground on accelerating Deep Neural Networks in some exciting application areas.
Graphcore’s IPU systems are enabling researchers at PNNL to reduce time to train for their AI models in the computational chemistry and cybersecurity domains, from days to hours. Besides demonstrating significant performance benefits on transformer-based models, IPU-POD systems are pushing the boundaries of innovation in emerging model architectures like Graph Neural Networks. This is helping PNNL incorporate groundbreaking machine learning tools into their research mission in meaningful ways.
“At Pacific Northwest National Laboratory, we are pushing the boundaries of machine learning and graph neural networks to tackle scientific problems that have been computationally challenging with existing technology,” said Sutanay Choudhury, Deputy Director of PNNL's Computational and Theoretical Chemistry Institute.
“For instance, we are pursuing applications in computational chemistry and cybersecurity applications. Our collaboration with Graphcore has allowed us to significantly reduce both training and inference times from days to hours for these applications. This speed up shows promise in helping us incorporate the tools of machine learning into our research mission in meaningful ways. We look forward to extending our collaboration with this newest generation technology.”
Accelerating Neural Networks for Quantum Chemistry applications
The application of machine learning techniques such as supervised learning and generative models in chemistry is an active research area.
ML-driven prediction of chemical properties and generation of molecular structures with tailored properties have emerged as attractive alternatives to expensive computational methods.
Improving the scalability of training these machine-learning models is essential to accelerate scientific discovery, and molecular datasets have unique computational characteristics for processing millions of small graphs with widely varying size and sparsity characteristics.
PNNL and Graphcore collaborated to demonstrate that the IPU’s fine-grained parallelism, coupled with a novel scheme for packing multiple small graphs into a single training sample, achieves state-of-the-art accuracy while reducing time to train by orders of magnitude compared to previously reported results.
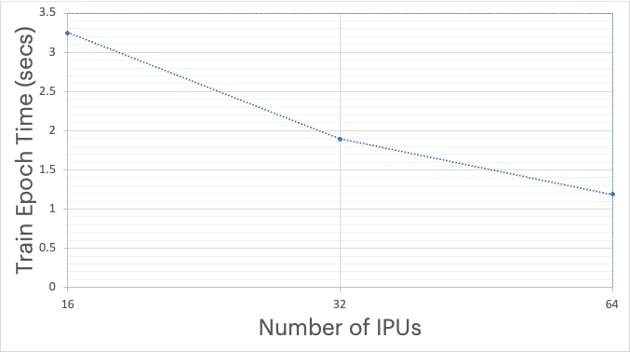
Figure 1: SchNet model trained on QM9 dataset using PyTorch Geometric
Cybersecurity applications on IPUs
Machine learning techniques are increasingly being used in the cybersecurity domain to improve software resiliency against security attacks and predict any potential areas of vulnerability. Classifying or mapping of known CVEs (Common Vulnerabilities and Exposures) to CWEs (Common Weakness Enumerations) provides a means to identify and mitigate vulnerabilities.
PNNL along with their academic collaborators have identified a novel transformer-based learning framework, called the V2W-BERT framework, that uses concepts from natural language processing, link prediction and transfer learning to classify vulnerabilities with greater accuracy and more effectively map weaknesses to exploits. Collaborators from Purdue University, PNNL and Graphcore came together and demonstrated excellent throughput/convergence results on IPUs and scalability going from 16 to 128 IPUs.
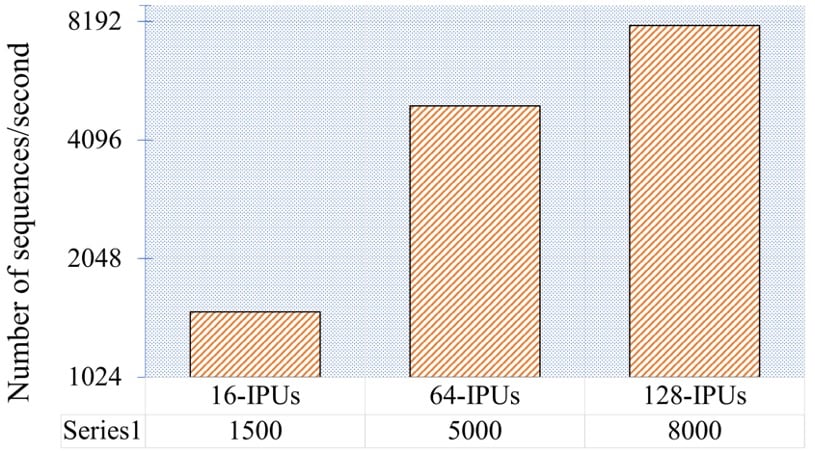
Figure 2 - Number of sequences per second processed on Graphcore IPUs (16, 64 and 128 IPUs) for BERT-V2W-Large pre-training with a sequence length of 128 tokens. The dataset consists of 137,225 sequences, resulting in epoch times of 91 seconds on 16 IPUs, 27 seconds on 64 and 17 seconds on 128 IPUs respectively.
The generalizability and scalability of the V2W-BERT framework will benefit both the theoretical development and practical deployment of novel cyber defense solutions and vulnerability classification.
In the future, the PNNL-Graphcore partnership will extend to several other areas to further advance the use of AI based techniques in scientific research. We are looking forward to the exploration of new frontiers in the use of AI for scientific research.
Thanks to: Sutanay Choudhury (PNNL), Jenna Pope (PNNL), Mahantesh Halappanavar (PNNL), Richie Singh, Saurabh Kulkarni