Graphcore’s latest submission to MLPerf demonstrates two things very clearly – our IPU systems are getting larger and more efficient, and our software maturity means they are also getting faster and easier to use.
Software optimisation continues to deliver significant performance gains, with our IPU-POD16 now outperforming Nvidia’s DGX A100 for computer vision model, ResNet-50.
Training ResNet-50 takes 28.3 minutes on the IPU-POD16, compared to 29.1 minutes for DGX A100 – a performance improvement of 24% since our first submission through software alone. It is a significant milestone, given that ResNet-50 has traditionally been a showpiece model for GPUs.
Our software-driven performance gain for ResNet-50 on the IPU-POD64 was even greater at 41%.
We also have results for our recently announced IPU-POD128 and IPU-POD256 scale out systems, which we entered straight into MLPerf’s ‘available’ category, reflecting Graphcore’s commitment to deliver outstanding performance at increasing scale.
For our larger flagship systems, time-to-train on ResNet-50 was 5.67 min on the IPU-POD128 and 3.79 mins on the IPU-POD256.
For the natural language processing (NLP) model BERT, we submitted on the IPU-POD16, IPU-POD64 and IPU-POD128, in both the open and closed categories, with an impressive time to train of 5.78 minutes on the new IPU-POD128, in our open submission.
Overall, we saw improvements for BERT performance of 5% for IPU-POD16 and 12% for IPU-POD64 when compared to the last MLPerf training round.
MLPerf’s Closed division strictly requires submitters to use exactly the same model implementation and optimiser approach, which includes defining hyperparameter state and training epochs.
The Open division is designed to foster innovation by allowing more flexibility in the model implementation while ensuring exactly the same model accuracy and quality is reached as the Closed division.
By showing results for BERT training in the Open division we are able to give a sense of the performance that Graphcore customers are able to achieve in the real world where they, naturally, take advantage of such optimisations.
Huge advantage at scale for new models
At Graphcore we are highly supportive of MLPerf and its organising body MLCommons. We understand that its third-party verification plays an important role in helping customers independently assess the capabilities of AI compute systems and the maturity of the software stacks provided by different companies.
While customers continue to use models like ResNet and BERT in production, they are also exploring innovative, new models and looking to Graphcore’s larger flagship systems to deliver machine intelligence at scale.
While not part of our MLPerf submission, innovative computer vision EfficientNet B4 trains in just 1.8 hours on our flagship IPU-POD 256 a massive, real-world performance advantage.
For customers who care about absolute throughput performance and scaling-out to our larger IPU-POD systems, we also see impressive results outside of MLPerf across a range of models including GPT-class models for natural language processing model and ViT (Vision Transformer) for computer vision.
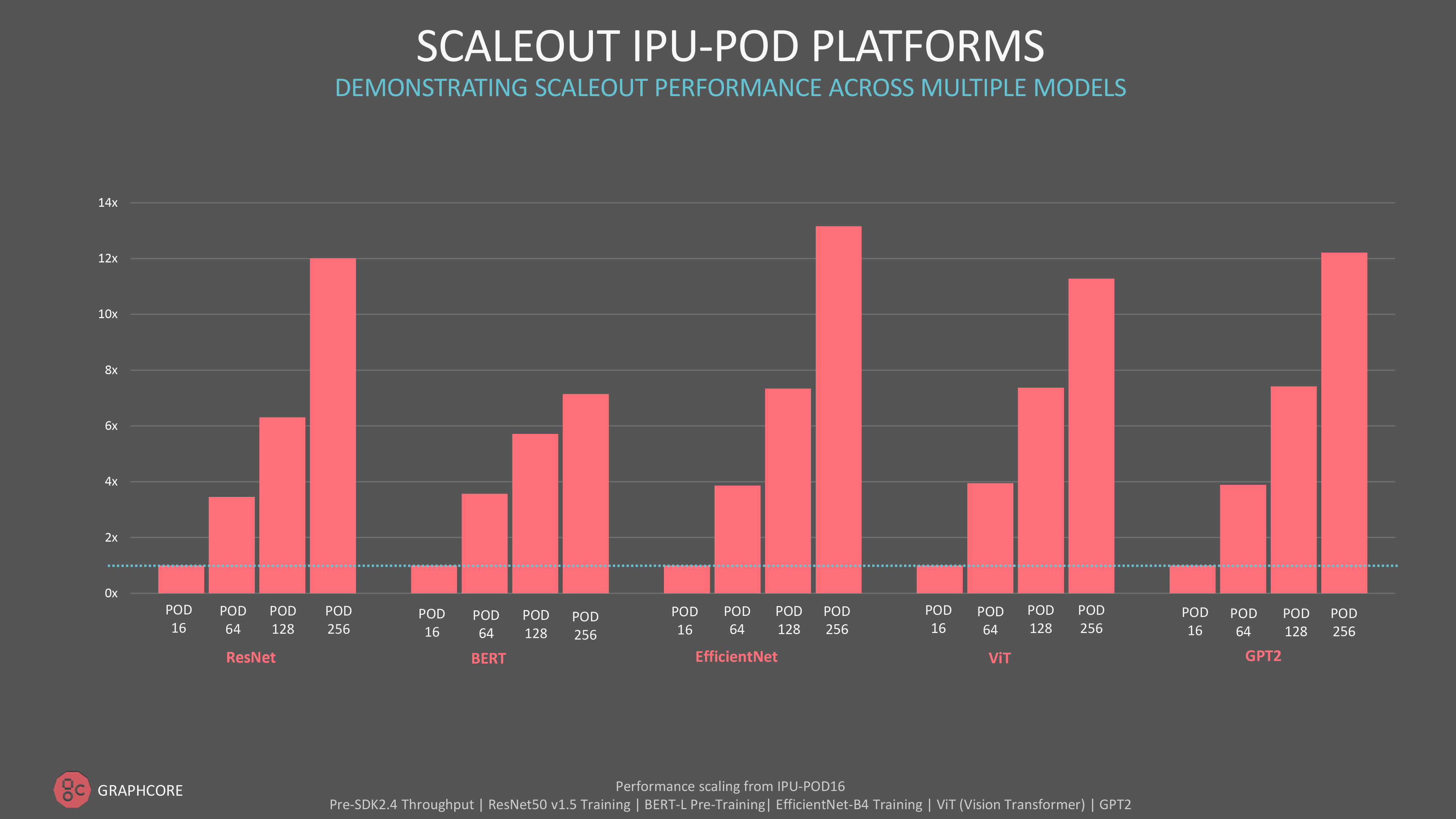
Efficient at scale by design
Anyone looking across the raw data for this, or any, MLPerf round will be struck by number of host processors associated with each manufacturer’s system, with some participants specifying one CPU for every two AI processors.
At the other end of the scale, Graphcore consistently has the lowest ratio of host processors to IPUs.
Unlike everyone else, the IPU only uses host servers for data movement and does not require host servers dispatching code at runtime. As a result, IPU systems need fewer host servers, resulting in more flexible and efficient scale out systems.
For a natural language processing model like BERT-Large, an IPU-POD64 only needs one dual-CPU host server. ResNet-50 requires more host processor support for image pre-processing, so we specify four dual-core servers per IPU-POD64. That ratio of 1-to-8 is still less than every other MLPerf participant.
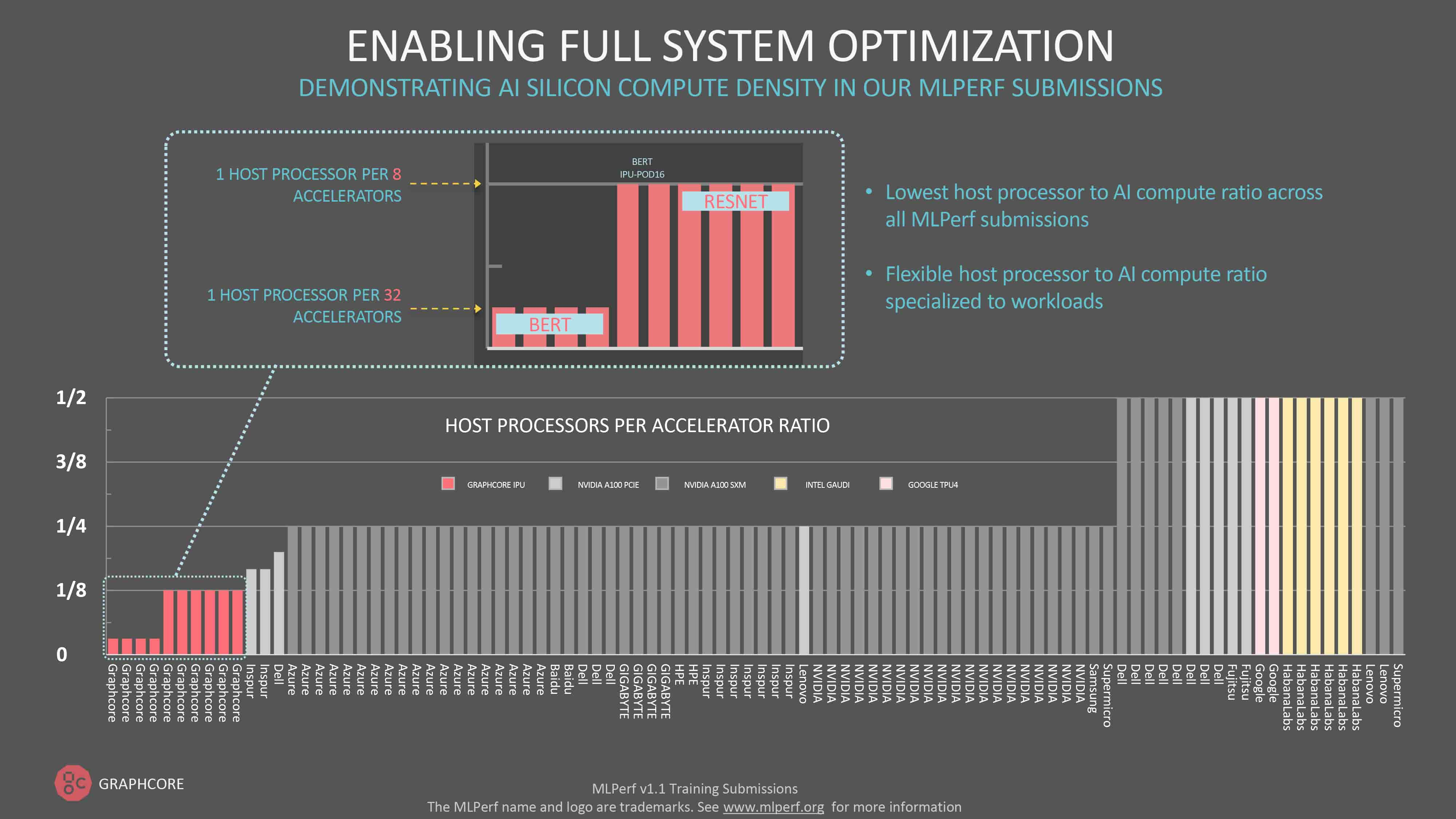
In fact, in this MLPerf 1.1 training round, Graphcore delivered the fastest single-server time-to-train result for BERT at 10.6 minutes.
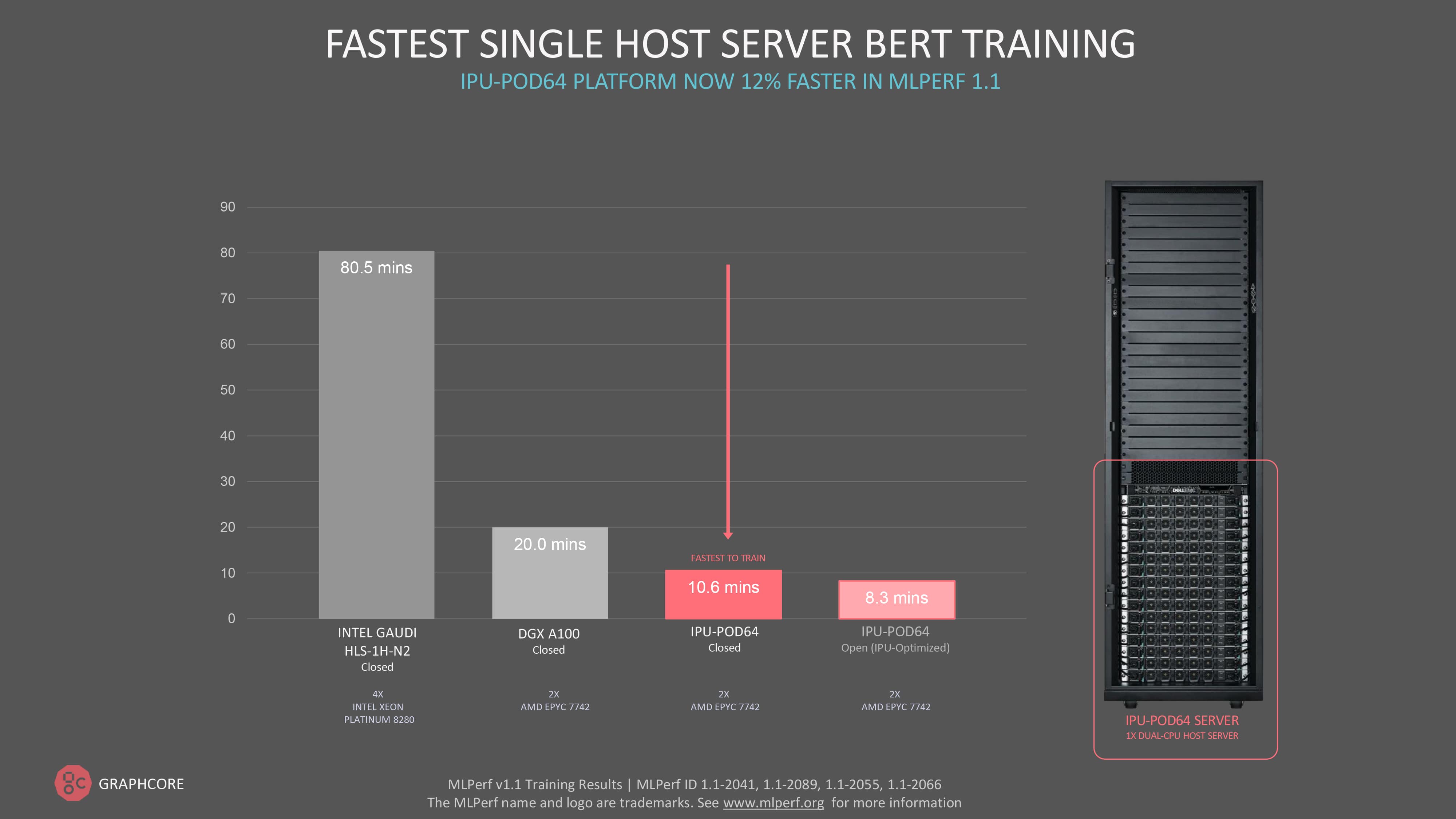
Relentless innovation
The combination of continuous optimisation to our Poplar SDK, and introduction of new IPU-POD products, has enabled a dramatic increase in the AI training capability that we are able to offer customers.
The tremendous progress seen since Graphcore’s first MLPerf submissions earlier in 2021, and indeed across the whole of this year, is testament to the company’s culture of relentless innovation.
This begins with the architectural choices that we make when designing our systems, such as the decision to disaggregate our host servers and AI compute, a fundamentally different approach to others in the industry, but one which is now proving its value.
Relentless innovation is also what drives us to push out major software updates at least every three months, with resulting performance gains, not to mention the work we do with customers, implementing and optimising new models and workloads for the IPU.
That enthusiasm is contagious. Throughout 2021, we have seen countless hardware and software partners – from Hugging Face and PyTorch Lightning to VMWare and Docker Hub - join Graphcore in supporting our goal of delivering the ultimate in AI compute performance on systems so easy to use that they are effectively accessible by anyone.
We’ve come so far in such a short time. Imagine where we will be 12 months from now.