In telecommunications today, machine intelligence, software-defined networking and 5G technologies are all combining to power a new era of data services and experiences for consumers.
Machine intelligence in particular will be crucial to the successful global rollout of 5G networks. Emerging applications such as smart cities and autonomous vehicles require consistent high bandwidth and low latency from 5G connectivity to operate safely and effectively. Machine intelligence can bring much needed predictability to 5G networks when the right machine learning models are applied. Both new and existing models will have their part to play in driving the 5G era forward, considering the complexity of today’s wireless networks.
With intelligent connectivity, telecommunications innovators can use AI to:
- Optimize network performance and manage network resources
- Forecast connectivity issues and automate repairs
- Enhance and personalize customer experience
- Deliver data services at scale for emerging 5G applications
Here are three examples of how machine intelligence will fundamentally change the future of networking:
Predictive Networks
Analyzing and predicting how a network will perform is becoming increasingly complicated for telecom operators. Increasing customer experience expectations are demanding even higher levels of service and reliability. Ideally, this would mean pre-empting and fixing faults before any service interruption occurs. But there are many challenges around predictability. Getting exact data is difficult, and noisy network performance data can significantly impact optimization and quality forecasting.
Machine intelligence is an invaluable tool for predicting performance as it is capable of learning from imperfect, noisy data, rather than relying on exact figures. Probabilistic time series models work especially well for forecasting network performance. Long short-term memory (LSTM) models are artificial recurrent neural networks which can be used for network traffic matrix prediction. By analyzing changes in wireless data, LSTM can determine performance patterns and predict future performance, facilitating network planning. As a highly complex model, it can equally take data uncertainty into account when predicting future performance.
LSTM’s complexity presents both an advantage and a disadvantage to the telecoms industry, however, since highly complex models are slow to run on legacy AI processors. The 5G networks of tomorrow require constant, real-time connectivity and cannot afford to sacrifice latency for complexity.
At Graphcore, we have optimized our Intelligence Processing Unit (IPU) to enable innovators to take advantage of state of the art performance on both existing and emerging models. Our IPU works especially well on highly complex models, driving significantly higher throughput at the same latency for LSTM inference microbenchmarking in comparison with leading alternative processors.
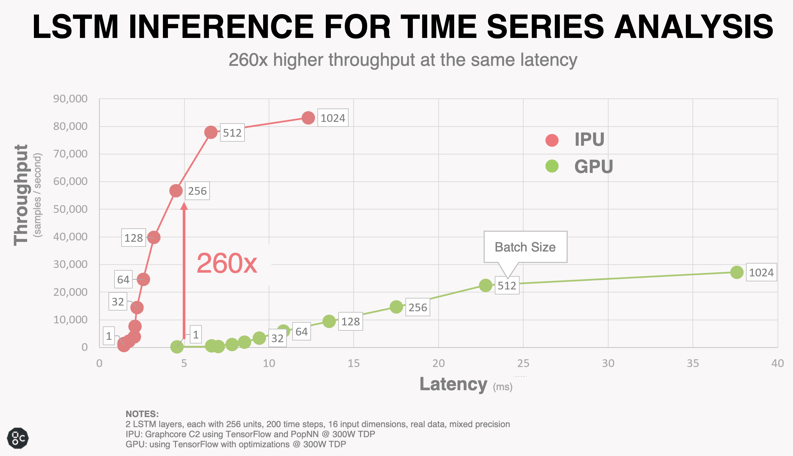
Intelligent 5G
Emerging applications such as connected cars, smart factories and augmented reality will rely heavily on consistent 5G connectivity. But meeting the right bandwidth and latency requirements for each of these use cases increases complexity and expense for telecom service providers.
One solution that is gaining traction is network slicing, which enables operators to distribute “slices” of network resources to different services, while controlling each slice’s functions and features. Machine intelligence adds further flexibility, adapting resource management to users’ activities for each slice. Different slices can meet different user requirements, providing the right bandwidth and latency whether users are on a conference call or streaming a film.
In terms of specific machine intelligence approaches, deep reinforcement learning can be used for network slicing and resource management. By learning from experience rather than interpreting pre-labelled data, deep reinforcement learning techniques can accurately identify network data in real time and match the right quality of service to the right applications.
Deep reinforcement learning models can unfortunately still take a long time to train on legacy processors, making them potentially less useful in real-world scenarios. By running deep reinforcement learning policies on an IPU instead, innovators can make use of the processor’s massive parallelism to bring new efficiency levels to applications where automated sequential decision-making is required such as network slicing. The example below shows a representative model which enables 13x higher throughput and faster time to train.
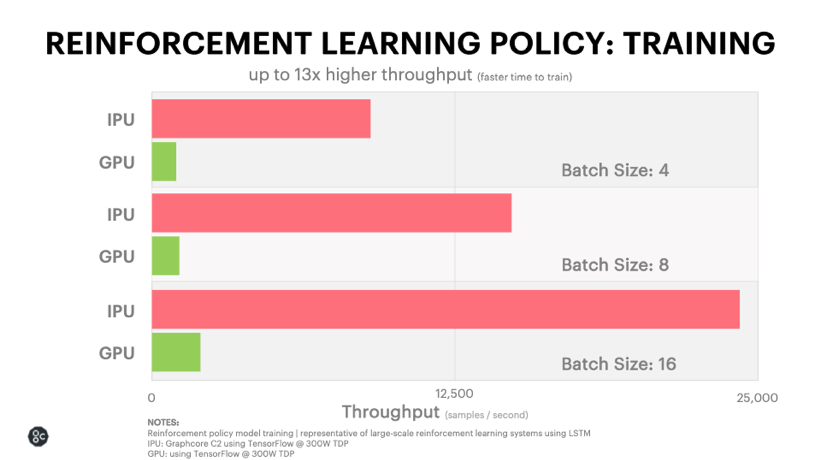
Customer Experience
Machine intelligence provides significant opportunities for service providers to personalize and enhance customer experience. Virtual assistants and chatbots are being used to automate many aspects of complaint handling and management, reducing costs and boosting efficiency while freeing up staff to work on more complex tasks.
Customers appreciate these assistants as well, as waiting times are reduced and complaints can be resolved much faster. All the data accumulated by the chatbot can then be pooled into the operator’s knowledge base in a standardized format, making data analysis much more straightforward. This approach helps to track ongoing performance issues, with the potential to feed this information back into predictive network models to improve their understanding of performance patterns.
In telecommunications, many are utilizing Natural Language Processing (NLP) models for innovation in customer experience. One relatively new NLP model that has been gaining traction for its state of the art performance in natural language understanding is Google’s Bidirectional Encoder Representations from Transformers (BERT), published in 2018. This unsupervised, bidirectional training enables vast improvements in accuracy for language understanding, resulting in a better experience for the user.
BERT has not been widely leveraged in NLP use cases in telecommunications so far, though its uptake would be a key differentiator for operators looking for intelligent solutions to gain a competitive edge in customer service. The challenge with integrating BERT is that it is a large-scale model requiring significant compute. Yet with the IPU, Graphcore has been able to demonstrate state of the art time to train with BERT-Base at 20% lower power than leading GPUs.
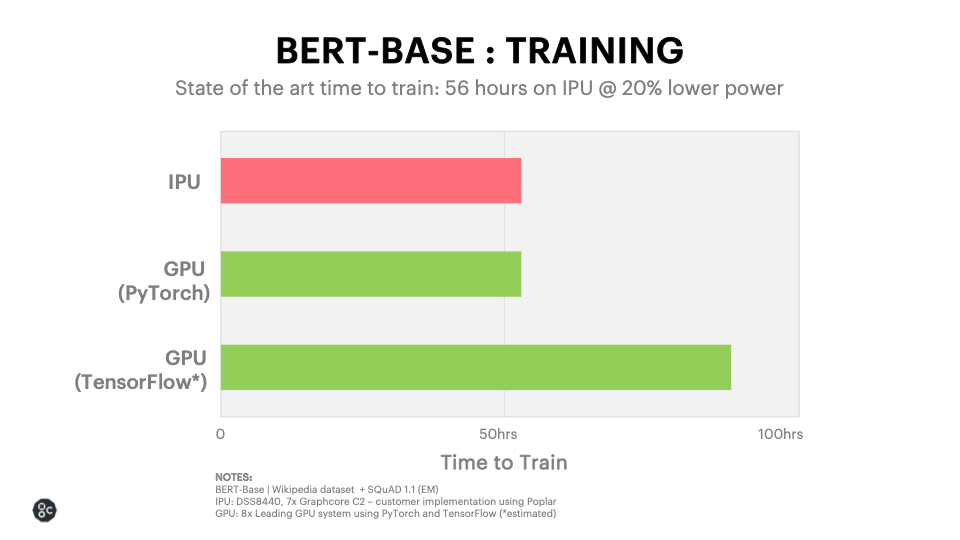
For BERT-Base inference, the IPU delivers 3x higher throughput at 30% lower latency.
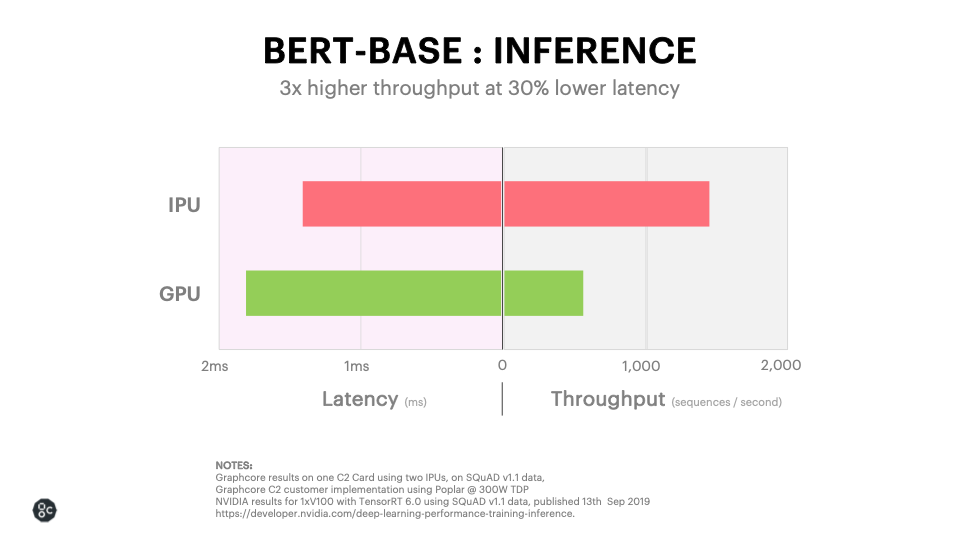
By combining the IPU’s state of the art processing power with new natural language processing breakthroughs, this performance advantage offers unprecedented customer data intelligence for industry innovators while boosting consumer satisfaction and loyalty.
The Future of Intelligent Connectivity
New developments in machine intelligence will continue to drive 5G network innovation forward, but it is worth remembering that processing challenges still remain, and that, as a result, the most suitable and most innovative models for network transformation are not always being harnessed.
To build the 5G networks of the future that will support new, data-centric applications and meet customers’ evolving expectations, telecoms innovators will need to leverage new and complex models, as well as traditional machine intelligence approaches.
Though many machine learning models already exist which will be invaluable in telecommunications implementations, there will be many more breakthroughs to come. By using processors that are designed to run machine intelligence workloads, operators will have the necessary compute to continue to innovate in the long term, even as new machine learning models are created.
Graphcore is accelerating machine intelligence to drive innovation forward in the telecommunications industry. If you are interested in finding out more about our telecom offering, get in touch with us here.